Breast Cancer Database Opens New Window on Deadly Disease
The effort was the culmination of collaboration between esteemed faculty
More than a quarter-million American women will be diagnosed with breast cancer this year.
“Although that figure hasn’t changed much year over year, the survival rate in older patients has continued to improve during the past decade,” says Seema Khan, MD, the Bluhm Family Professor of Cancer Research and a member of the Northwestern University Clinical and Translational Sciences (NUCATS) Institute and Robert H. Lurie Comprehensive Cancer Center of Northwestern University. “Research continues to build upon itself as we learn more and more about the disease, how to treat it, and how to prevent it.”
A new dataset — the result of a collaborative project between Khan and NUCATS Institute Chief AI Officer Yuan Luo, PhD — has made the process of reviewing thousands of patient histories increasingly possible.
The process relies on machine learning to mine the electronic health records of nearly 10,000 breast cancer cases in an effort to deliver new data to researchers.
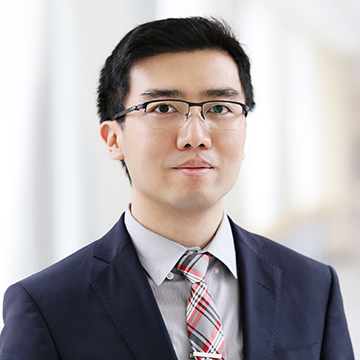
This was a multiyear effort started in 2016 and spearheaded by our joint PhD student Zexian Zeng, and represents an exemplary and seamless collaboration between research teams led by Dr. Khan and myself.”
Yuan Luo, PhD,NUCATS Institute Chief AI Officer and associate professor of Preventive Medicine in the Division of Health and Biomedical Informatics
"This was a multiyear effort started in 2016 and spearheaded by our joint PhD student Zexian Zeng, and represents an exemplary and seamless collaboration between research teams led by Dr. Khan and myself,” says Luo, associate professor of Preventive Medicine in the Division of Health and Biomedical Informatics and a member of the Robert H. Lurie Comprehensive Cancer Center of Northwestern University.
The researchers mined structured information from unstructured clinical notes and provided access to these structured data through the Northwestern Medicine Enterprise Data Warehouse.
“We used natural language processing to identify the related information in hundreds of thousands of clinical notes of nearly 10,000 breast cancer patients,” says Luo. “This information was then painstakingly reviewed by two annotators who confirmed the data by chart-reviewing the notes.”
Several of Khan’s breast cancer research colleagues have also used the dataset, which has been validated by numerous peer-reviewed publications. In one study, researchers extracted features from clinical notes and also retrieved structured clinical data by training a program to identify distant recurrences in breast cancer patients. Accurately identifying distant recurrences — the spread of cancer — in breast cancer from electronic health records is important for both clinical care and secondary analyses. Although multiple applications were previously developed, distant recurrence identification still relied heavily on manual chart review, until now.
This has been extremely well done in terms of the tumor information and biological information entered. The fact that we have thousands of vetted patient records is a big deal, but we also know that we can continue to improve."”
Seema Khan, MD, the Bluhm Family Professor of Cancer Research and a member the NUCATS Institute and Robert H. Lurie Comprehensive Cancer Center of Northwestern University.
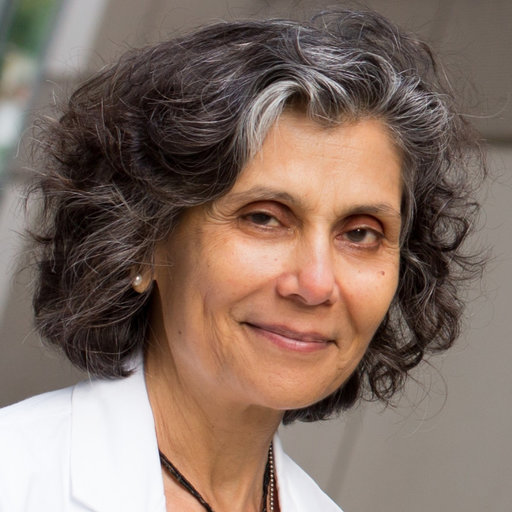
“We aimed to develop a model that identifies distant recurrences in breast cancer using clinical narratives and structured data from electronic health records,” says Luo, corresponding author of the study. “The resulting information can help accurately and efficiently identify distant recurrences in breast cancer by combining features extracted from unstructured clinical narratives and structured clinical data.”
A second study focused on using natural language processing and machine learning to identify local recurrences of breast cancer. Compared with labor-intensive chart review, the new model used the dataset to create an automated approach for the process.
“The collaboration to establish this dataset worked well and involved Dr. Luo’s team enhancing natural language processing algorithms and my team reviewing and refining the data,” says Khan, a physician-scientist who specializes in surgical treatment for women with breast cancer. “Our team verified that we could extract the truth by helping to refine the algorithm to get accurate data.”
Members of the Northwestern University Feinberg School of Medicine and Lurie Cancer Center have accessed the database, and Luo and Khan hope to add more health records in the future.
“This has been extremely well done in terms of the tumor information and biological information entered,” says Khan. “The fact that we have thousands of vetted patient records is a big deal, but we also know that we can continue to improve.”
Written by Roger Anderson